The 2nd International Conference for CBM in Aerospace, which included the MORPHO mid-term conference on its first day, took place on Wednesday, September 11, 2024, in Paris at ENSAM University.
The event commenced with the Opening Ceremony, chaired by Nazih Mechbal, our project coordinator and the director of the Processes and Engineering in Mechanics and Materials Laboratory at Arts et Métiers (Paris). Professor Mechbal welcomed the participants and set the tone for the conference. The initial speech was delivered by Professor Fu-Kuo Chang from the MORPHO Advisory Board (Stanford University, USA), focusing on "Structural Health Monitoring for Condition-Based Maintenance."
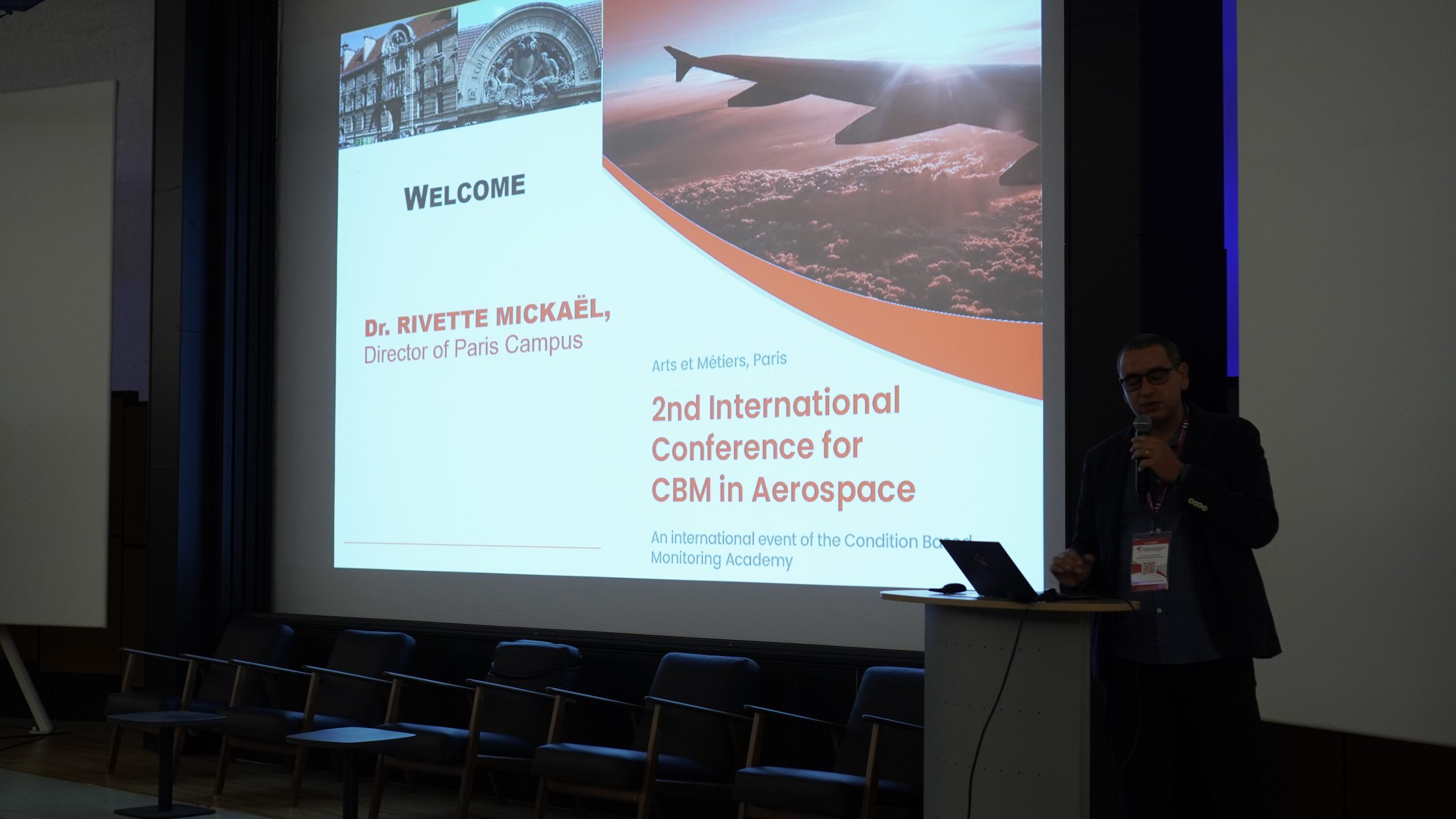
In the afternoon, the S5 session focused on MORPHO, featuring presentations from consortium members on "Embedded Life-Cycle Management for Smart Multimaterials Structures." This session was chaired by Dr. Theodoros Loutas, an Associate Professor at the Department of Mechanical & Aeronautical Engineering at the University of Patras, and Nazih Mechbal.
On this page, you'll find a gallery of event photos, a video summary, and abstracts of each presentation from the Morpho session. Feel free to explore!
Missed the MORPHO midterm conference? Catch the highlights in our video summary!
Real-Time RTM Process Monitoring: Detection of the Resin Flow Front using FBG Sensors
Authors: Andreas Krenz, Vladislav Reimer, Jan Koch
Abstract : The aerospace industry continually seeks advancements in materials and manufacturing processes to enhance the performance and safety of ... aircraft components.
This study presents an advanced process monitoring approach for Resin Transfer Molding (RTM) applied to a hybrid material composed of carbon fiber reinforced plastic (CFRP) and metal reinforcement, developed for future applications for aircraft turbine blades. Fiber Bragg Grating (FBG) sensors are directly integrated into the CFRP preform, enabling to monitor relevant process parameters such as resin flow and temperature inside the component during the manufacturing process.
The accurate detection of the resin flow front is crucial for preventing dry spots and ensuring the complete infusion of the preform, which directly impacts the mechanical properties of the final part.
To interpret the complex data from the FBG sensors, a novel data evaluation method has been developed to identify the resin flow movement profile during the RTM process.
This evaluation method enables the identification of flow front anomalies and can help to optimize RTM process parameters. The evaluation method is based on the detection of a specific response behavior of the FBG sensors, which is caused by the resin flow front passing through during the process.
One of the major challenges the signal processing which must enable differentiation flow front detection from other influences during the RTM process, such as the influence of the vacuum. Figure 2 shows a visualization of the resin flow front arrival based on the automatically evaluated FBG data.
Furthermore, the FBG sensor data are validated by cross-comparison with resistance sensors data that detect the resin at several locations in the RTM mold to ensure a reliable process monitoring system. The produced components equipped with embedded FBG sensors are then subjected to load tests to develop suitable SHM strategies using the strain data gathered from the measurements of the identical FBG sensors used for RTM process monitoring.
The study demonstrates that the integration of FBG sensors into the CFRP preform offers bifunctional benefits: It offers precise real-time monitoring of the resin flow front during the manufacturing process and subsequently allows monitoring of the structural condition of the component during the service life. This enables predictive maintenance strategies that potentially extend the life of the turbine blade and increase safety.
The proposed sensing concept may represent the next generation in health management and monitoring of smart structures during both the production stage and further operation.
Andreas Krenz, Fraunhofer IFAM

Hybrid Twin of the Resin Transfer Molding Process
Authors: Eric Monteiro, Sebastian Rodriguez Iturra, Nikos Pantelelis, Alfredo De Gregorio De Juan, Adrien Touze, Marc Warris, Marc Rebillat, Francisco Chinesta, Nazih Mechbal
Abstract : The Resin Transfer Molding (RTM) process is widely used for manufacturing composite parts in aeronautic but is still not robust. ... One objective of the MORPHO project is to develop a strategy to optimise in real-time the RTM process. To do so, new sensors have been developed as well as new hybrid model of the process. This model combines a reduced multiphysics finite element model and a data-driven ignorance model to predict in real-time the resin flow front position.
Printing of piezoelectric and temperature sensors on composite panels
Authors: Ingo Wirth
Abstract : In aviation industry, there exists an increasing demand for structural health monitoring (SHM) of carbon fiber reinforced composite materials (CFRP), ... which are needed for aerospace structures because of their unique stiffness to weight ratio.
The challenge in such a context is to integrate smart systems in composites for lightweight constructions using different sensors without mechanically changing the structural behavior of the host structures (low weight addition and as small as possible stiffness modification).
Innovative printing technologies allow the integration of printed sensors in composite parts and components by satisfying these criteria. For this purpose, manufacturing and integration process of sensors in composite parts using printing technologies was investigated. Piezoelectric sensors (PZT) as well as temperature sensors were deposited directly on composite aeronautics parts representative of the aeronautic industry using screen printing and Aerosol Jet printing technologies.
FBG-Based Structural Health Monitoring of Engine Blades Towards Intelligent Structures and CBM
Authors: George Galanopoulos, Shweta Paunikar, Marc Rebillat, Dimitrios Zarouchas
Abstract: Structural Health Monitoring (SHM) has been gaining increased attention over the past decades as an important step towards Condition ... Based Maintenance (CBM).
Measurements from the SHM systems provide the necessary information to monitor the condition of a (sub)component or structure and enable the use of this knowledge for maintenance task when needed, increasing availability and safety while reducing downtime related costs.
On the final level of SHM lie diagnostics and prognostics, whose output inform about the current and future state of the (sub)component and their accuracy impact the effectiveness of the CBM decision making, and hence a capable sensor network is important. In this research our focus lies with monitoring the structural integrity of composite aircraft engine blades, through a capable network of SHM technologies. Engine blades are an important part of any aircraft and their integrity is imperative for its safe operation.
A common yet critical damage case is impact damage (cause by hail or bird strikes) which can significantly reduce the load bearing capabilities of the blade. The aim is to demonstrate the feasibility, effectiveness and usefulness of different SHM systems in identifying the existence of damage, monitoring the damage and degradation growth and eventually use robust and reliable indicators to estimate the remaining useful life. To accomplish that task, a subpart of the engine blade is manufactured from 3D-woven CFRP preforms via the injection molding technique. The panels are curved and their length is 800mm while their width is 350mm.
A secondary adhesively bonded steel edge is also adhered to the entire length of the panel with a width of 50mm. The panels are subjected to a repeated 4-point bending load-unload scheme with increased severity to simulate low frequency fatigue at ~0.02 Hz and introduce controlled and gradual degradation. First, one panel is subjected to 4-point bending quasi-static loading to determine the failure load which was approximated at 22kN through finite element analysis. Experimental collapse was reached at 28 kN and with this a guide the load envelope was decided. The loads include [4, 8, 12, 14, 16, 18, 20, 22, 24, 26, 28] kN and each load is applied for 400 cycles. Impact damage is also introduced to most of the panels in order to create a damage area to monitor.
Regarding the SHM systems employed, the panel is equipped with state of the art FBG sensors and piezoelectric sensors. In this work, the data from the optical fibers are studied more in depth. The fibers can either be surface mounted (tensiled side of the panel) or embedded during the layup process or a combination of both. The majority of optical fibers run across the length of the panel at different width locations, two focused in the middle section, and one close to each of the edges. The sensors are used to monitor the strain field and it is attempted to correlate damage formulation and overall degradation with alterations to the strain field. The first indication of degradation can be observed by analyzing the data collected from the MTS (the hydraulic machine used to perform the experiments).
By processing the load and displacement data, the experimental stiffness can be calculated as the slope of a linear equation between the load and displacement during the loading part. What was observed, is that at first the experimental stiffness slightly increases after the first load case, attributed to the increased robustness of the panel after significant bending. A somewhat constant stiffness follows, until the time close to failure, where rapid stiffness drop can be seen. This is accompanied by the first visible mode of damage in the form of skin tears and fiber cracking at the top surface close to the loading pins. Final collapse is dominated by matrix and fiber breakage close to the loading pin locations which extend across the entire width of the panel.
These results are summarized in Figure 1 and Figure 2. FBG data are dependent on the type of FBG. Embedded FBG sensors display a mixture of tension and compression behavior while surface mounted show predominantly tension strains with increased intensity as the max load increases.
The end goal of analyzing the FBG data is to extract capable indicators, similar to, and correlate the online SHM measurements to the degradation, as observed by the stiffness reduction, in a semi-quantitative way. In this research the use of strain based SHM sensors for degradation monitoring is demonstrated. Strain based indicators were used in an attempt to capture degradation evolution in large curved composite panels representative of aircraft engine blades.
An unprecedented experimental campaign was launched on engine blade panels, which are subjected to fatigue-like 4-point bending load, and are mounted with state of the art SHM systems. The raw strains are transformed into an informative measure of degradation, demonstrating the feasibility, effectiveness and usefulness of such sensors for diagnostic and prognostic tasks, a first step towards a CBM paradigm.
Using Novel Printed Piezoelectric Sensors for Monitoring the Health of a Composite Foreign Object Damage Panel
Authors: Shweta Paunikar, George Galanopoulos, Marc Rebillat, Ingo Wirth, and Nazih Mechbal.
Abstract : This research focuses on the structural health monitoring (SHM) of a foreign object damage (FOD) composite panel substructure of an ... aircraft engine fan blade equipped with an architecture array of novel screen printed piezoelectric sensors and is being carried out within the purview of the MORPHO – H2020 project.
The state of the art printing technology ensures that architectured network of printed sensors is not only non-intrusive and lightweight but can also be printed during the manufacturing process before the structure goes into service. The FOD panel in this work is made of 3D woven composite, measuring approximately 800 mm x 350 mm, with a stainless-steel leading edge bonded to one of the longer edges and hosts a network of 5 arrays of 5 printed sensors each.
The printed sensors can potentially be used in multiple ways to analyse the health of the host structure. Since the fabrication process of the sensors is an on-going research, first, the electromechanical behaviour of the sensors is analysed with the help of impedance measurements.
It is observed that the printing process ensures repeatability. Secondly, the performance of the printed sensors in case of impact loading is discussed here, as bird impact is one of the leading causes of engine fan blade failure. The impact response measured by the printed sensors is studied to detect the impact location on the FOD panel. Next, the ability of the printed sensors to sense ultrasonic guided wave responses generated by standard ceramic piezoelectric disc actuators is demonstrated. Finally, the health of these printed sensors upon undergoing multi-load multi-cycle bending tests is also discussed here.
The ultimate goal of this project is to create diverse diagnostic and prognostic techniques for estimating the remaining lifespan and Structural Health Monitoring (SHM) of the FOD panel based on the range of measurements gathered using the printed sensors.
A DeepLearning based pipeline for online Remaining Useful Life prognostics
Authors: Giannis Stamatelatos, Theodoros Loutas
Abstract : The stochastic nature of composite structures poses a significant challenge for deriving analytical models to predict remaining useful life (RUL). ... Consequently, a data-driven approach becomes imperative, particularly when dealing with real-time operation scenarios.
Previous works employing Deep Learning require extensive data preprocessing, manual feature engineering, and the imposition of mathematical conditions (e.g., monotonicity), which pose limitations and drawbacks in the process. To address this issue, this study proposes a novel approach to RUL estimation, allowing the AI model to predict RUL online as new data points become available.
Leveraging sensor data acquisition techniques and pretrained models, we exploit the inherent capabilities of Deep Learning to extract patterns from highly nonlinear and complex data relationships, acknowledging the sequential nature of the data which inherits memory.
Our methodology involves preprocessing the raw sensor data and feeding it into the AI model, which automatically extracts relevant features without the need for extensive manual feature engineering. This streamlined pipeline enables fast and easy adaptation to new datasets and different problems, significantly reducing the preprocessing overhead. Through extensive experimentation and validation, our research demonstrates the effectiveness of this data-driven approach in accurately estimating the RUL of composite structures undergoing fatigue.
We thoroughly tested various stateof- the-art Deep Learning models and identified the most efficient and capable one (i.e. 1D Inception Convolutions) for our application. Despite the relatively small number of specimens used, we achieved a good validation and test metric of the predictions. This work emphasizes the novelty of enabling online prediction and the efficiency of the proposed pipeline in handling diverse datasets without requiring extensive preprocessing.
In conclusion, our study contributes to the advancement of predictive maintenance strategies, offering a practical and efficient solution for estimating the operational longevity of composite components in various engineering applications.
Theodoros Loutas, U. Patras
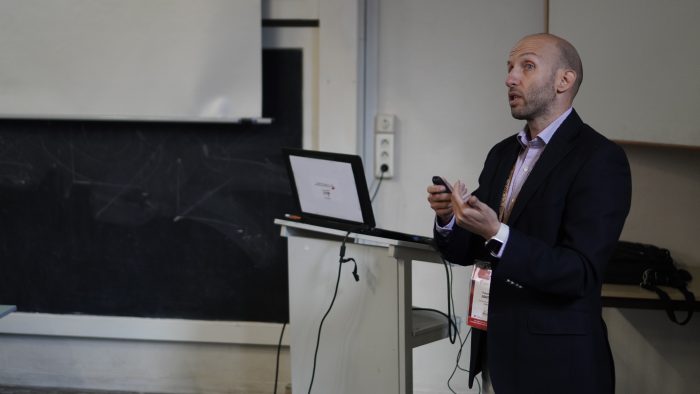